Top Fraud Email Datasets for Enhanced Fraud Detection and Prevention
Fraud email datasets are collections of emails that have been identified as fraudulent or scam attempts. These datasets are used for various purposes, such as training machine learning models to detect and prevent email fraud, analyzing patterns and techniques used by scammers, and developing algorithms to identify and filter out fraudulent emails.

US Consumer Demographics | Homeowners & Renters | Email & Mobile Phone | Bulk & Custom | 255M People
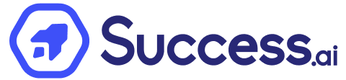
B2B Email Data | US Financial Services | Verified Profiles & Key Contact Details | Best Price Guaranteed
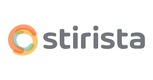
Identity Graph Data | 1.8 billion Consumer Email database to power Identity Graph, Identity Linkage, and Customer Recognition

Factori Identity Data | One Billion+ Identity Linkages, Validation and resolution Data|

Identity Linkage Data | MAID to HEM and FLIP | Global
Related searches

B2B Data | Company Data | TOP#1 Database: 360 Million Businesses Worldwide
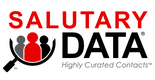
AI & ML Training Data | 148MM+ U.S Identities for Model Training | Identity Resolution | Identity Verification

Phone Number Data | USA Coverage | 765 Mil+ Numbers

Asia Pacific B2C Consumer Contact Lookup - Privacy-Compliant Identity Resolution

Residential Phone Number Data | USA Coverage | 74% Right Party Contact Rate | BatchData
1. What are fraud email datasets?
Fraud email datasets are collections of emails that have been specifically curated and labeled to contain instances of fraudulent or malicious activities. These datasets are used by researchers, data scientists, and organizations to develop and train machine learning models for fraud detection and prevention.
2. Why are fraud email datasets important for fraud detection and prevention?
Fraud email datasets play a crucial role in developing effective fraud detection and prevention systems. By analyzing these datasets, researchers and organizations can identify patterns, characteristics, and indicators of fraudulent emails. This knowledge can then be used to build robust algorithms and models that can automatically detect and flag potential fraud attempts.
3. Where can I find the best fraud email datasets?
There are several reliable sources where you can find high-quality fraud email datasets. Some popular platforms and repositories include Kaggle, UCI Machine Learning Repository, and the Enron Email Dataset. These platforms provide a wide range of datasets that can be used for fraud detection research and development.
4. What factors should I consider when choosing a fraud email dataset?
When selecting a fraud email dataset, it is important to consider factors such as dataset size, diversity, and labeling accuracy. A larger dataset with diverse examples can help improve the performance of your fraud detection model. Additionally, ensure that the dataset has accurate labels indicating fraudulent or non-fraudulent emails to ensure reliable training and evaluation of your models.
5. Can I use publicly available fraud email datasets for commercial purposes?
The usage rights and licenses of fraud email datasets may vary depending on the source. While some datasets may be freely available for both academic and commercial use, others may have specific restrictions. It is important to carefully review the terms and conditions associated with each dataset to ensure compliance with any usage restrictions or licensing requirements.
6. How can I evaluate the performance of my fraud detection model using these datasets?
To evaluate the performance of your fraud detection model, you can use various metrics such as accuracy, precision, recall, and F1 score. Split the dataset into training and testing sets, train your model on the training set, and then evaluate its performance on the testing set. Comparing the model’s predictions with the true labels in the testing set will give you insights into its effectiveness in detecting and preventing fraud.