What is Alternative Data? Examples, Providers & Datasets to Buy
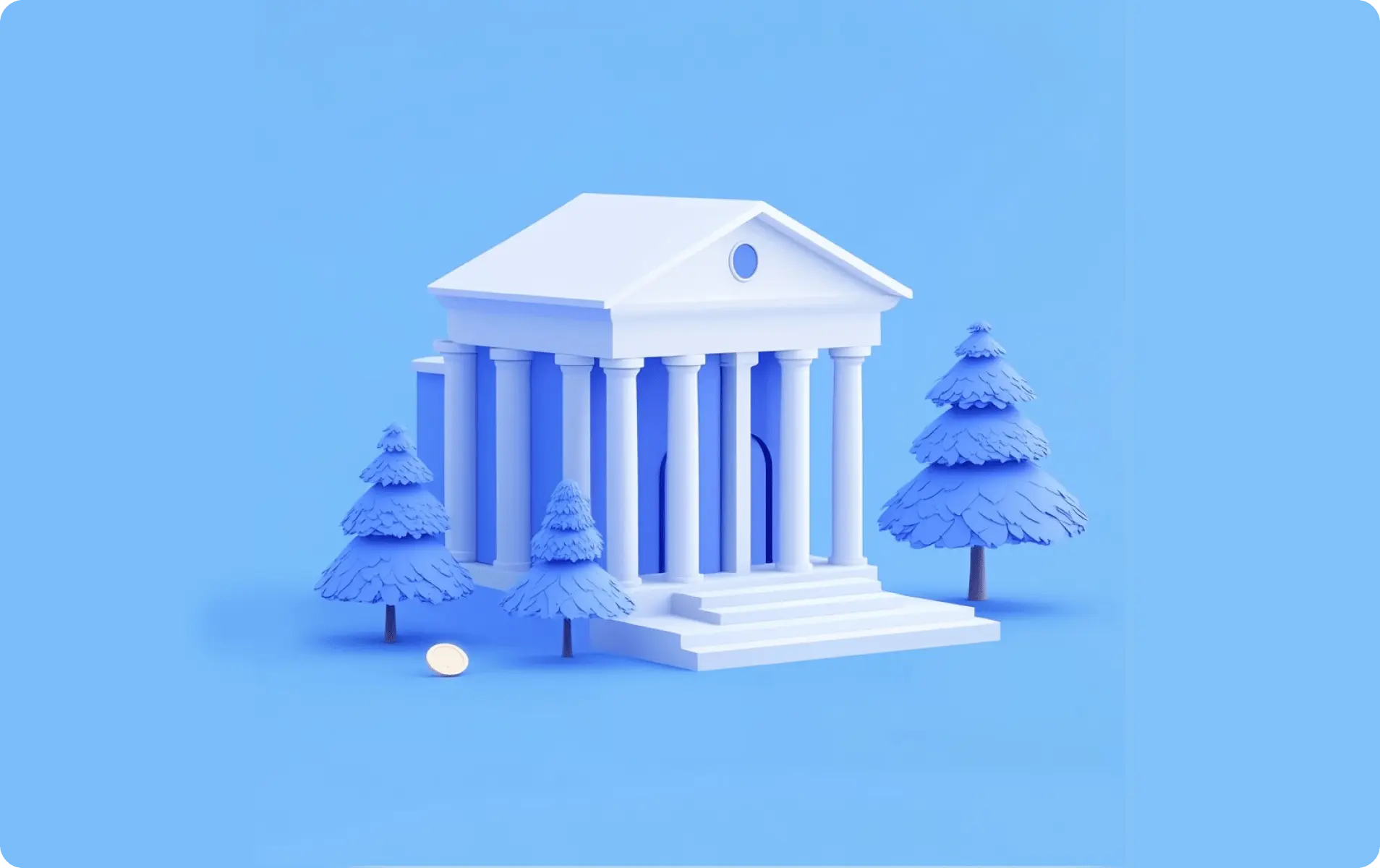
What is Alternative Data?
Alternative data refers to non-traditional sources of information used to gain insights and make informed decisions. It includes data from social media, satellite imagery, web scraping, credit card transactions, and more. This data complements traditional sources like financial statements and market reports, providing unique perspectives and uncovering hidden trends. Alternative data is increasingly utilized by businesses, investors, and researchers to enhance their understanding of markets, consumer behavior, and various industries.
What Are Examples of Alternative Data?
Examples of alternative data include datasets offering unique insights into consumer behavior, business performance, and market activity. Key examples include:
- Web Traffic Data: Visitor statistics, bounce rates, and engagement metrics for websites.
- Satellite Imagery: Visual data of agricultural fields, urban development, or shipping ports.
- Social Media Data: Posts, mentions, and sentiment analysis from platforms like Twitter and Instagram.
- Credit Card Transaction Data: Aggregated and anonymized consumer spending insights.
- Foot Traffic Data: Data on visitor patterns and movements around physical locations.
Editor's Pick
Best Alternative Data Databases, Datasets & API
The best alternative datasets provide innovative insights from unconventional sources, supporting analysis and decision-making across industries. This curated list features the top datasets and APIs, selected for quality, accuracy, and trusted providers where you can buy alternative data.

Alternative Data | Web Scraping | Custom Datafeeds | Consumer Data | Commerce Data
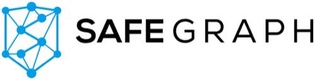
Alternative Data | B2B Location Data | SafeGraph Places
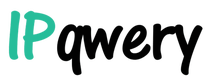
Alternative Data | KYB Data | Patents & Trademarks | +10M records | weekly updates

Coresignal | Company Financial Data | Alternative Data | Global / 1B+ Records / Largest Professional Network / Updated Daily

Point of Interest (POI) Data | 230M+ Locations | Global GIS Data | 3x Fresher Data, Alternative Data for Location Intelligence

Custom Data-Driven Market Research for B2B and B2C companies (Big Data + Machine Learning + Alternative Data Methodology)

Consumer Edge Transact Signal EUR Consumer Alternative Data | Austria, France, Germany, Italy, Spain, UK | 6.7M Accounts, 5K Merchants, 600 Companies

Rappi E-Receipt Data | Food Delivery Transactions (Alternative Data) | Latin America | Granular & Aggregate Data available

Global Web Scraping Data | Bespoke Alternative Data | Cleaning and Standardisation | Frequent Updates

Woodseer ETF Data - ETF Data, Alternative Data, Derivatives Data, Dividend Data and Dividend Forecast
Monetize data on Datarade Marketplace
Top Alternative Data Providers & Companies
Popular Use Cases for Alternative Data
Investors use alternative data for predictive modeling, leveraging unique data sources to forecast market trends and stock performance. Alternative data supports market analysis, offering granular insights into consumer behavior and business dynamics. Additionally, risk assessment uses alternative data to evaluate potential disruptions or opportunities in specific industries.
Main Attributes of Alternative Data
Below, we outline the most popular attributes associated with this type of data—features that data buyers are actively seeking to meet their needs.
Attribute | Type | Description | Action |
---|---|---|---|
String | The name of a company or business, might be the legal or brand name. | View 38 datasets | |
String | The ticker symbol of a stock. | View 31 datasets | |
String | The country code in Alpha-2 format (ISO 3166) | View 23 datasets | |
String | The name of a city. | View 14 datasets | |
String | The email address of a company or contact. | View 14 datasets | |
String | The name of an organization. | View 13 datasets |
Alternative Data Use Cases in Detail
Use Case 1: Investment Research and Analysis
Alternative data is widely used in investment research and analysis to gain insights into various industries and companies. By analyzing alternative data sources such as social media sentiment, web scraping, satellite imagery, credit card transactions, and more, investors can make more informed decisions about their investment strategies. This data can provide valuable information about consumer behavior, market trends, and company performance, helping investors identify potential investment opportunities and risks.
Use Case 2: Risk Management and Fraud Detection
Alternative data is also utilized in risk management and fraud detection across various industries. By incorporating alternative data sources into risk models, organizations can better assess and mitigate potential risks. For example, credit card companies can analyze transaction data to identify fraudulent activities and prevent financial losses. Insurance companies can leverage alternative data to assess the risk profile of policyholders and determine appropriate premiums. By utilizing alternative data, organizations can enhance their risk management practices and improve fraud detection capabilities.
Use Case 3: Supply Chain Optimization
Alternative data plays a crucial role in supply chain optimization by providing real-time insights into various aspects of the supply chain. Companies can leverage alternative data sources such as IoT sensors, weather data, and social media trends to monitor and predict demand patterns, optimize inventory levels, and improve logistics operations. By analyzing alternative data, organizations can make data-driven decisions to streamline their supply chain processes, reduce costs, and enhance overall operational efficiency.
Main Attributes of Alternative Data
Alternative data refers to non-traditional information that is used to gain insights and make informed decisions. It can be sourced from a variety of alternative data sources such as social media, satellite imagery, web scraping, credit card transactions, and more. The attributes of alternative data can vary depending on the specific dataset, but some common attributes include time-stamped data, unstructured data, high volume and velocity, diverse data types, and the ability to provide real-time or near real-time information. These attributes enable alternative data to offer unique perspectives and complement traditional data sources. Here’s a table of the main attributes you might find on Alternative Datasets:
Attribute | Description |
---|---|
Unconventional | Alternative data refers to non-traditional data sources that are not typically used in traditional financial analysis. |
Large Volume | It involves vast amounts of data, often in the form of unstructured or semi-structured data, which requires advanced data processing techniques. |
Variety | Alternative data encompasses a wide range of data types, including social media posts, satellite imagery, web scraping, credit card transactions, sensor data, and more. |
Timeliness | It provides real-time or near-real-time data, allowing for more up-to-date insights and faster decision-making. |
Granularity | Alternative data can offer detailed and specific information at a granular level, enabling more precise analysis and identification of trends. |
Non-consensus | It offers unique and differentiated insights that are not readily available through traditional data sources, potentially leading to a competitive advantage. |
Correlation Potential | Alternative data can reveal correlations and relationships that may not be apparent through traditional data sources, providing new perspectives for analysis. |
Data Privacy Concerns | Due to the nature of alternative data sources, privacy concerns and compliance with data protection regulations must be carefully addressed. |
Data Quality Challenges | Ensuring the accuracy, reliability, and consistency of alternative data can be challenging, as it often requires data cleansing and normalization processes. |
Data Integration Complexity | Integrating alternative data with existing data infrastructure and systems can be complex, requiring specialized tools and expertise. |
Frequently Asked Questions
Where Can I Buy Alternative Data?
You can explore our data marketplace to find a variety of Alternative Data tailored to different use cases. Our verified providers offer a range of solutions, and you can contact them directly to discuss your specific needs.
How is the Quality of Alternative Data Maintained?
The quality of Alternative Data is ensured through rigorous validation processes, such as cross-referencing with reliable sources, monitoring accuracy rates, and filtering out inconsistencies. High-quality datasets often report match rates, regular updates, and adherence to industry standards.
How Frequently is Alternative Data Updated?
The update frequency for Alternative Data varies by provider and dataset. Some datasets are refreshed daily or weekly, while others update less frequently. When evaluating options, ensure you select a dataset with a frequency that suits your specific use case.
Is Alternative Data Secure?
The security of Alternative Data is prioritized through compliance with industry standards, including encryption, anonymization, and secure delivery methods like SFTP and APIs. At Datarade, we enforce strict policies, requiring all our providers to adhere to regulations such as GDPR, CCPA, and other relevant data protection standards.
How is Alternative Data Delivered?
Alternative Data can be delivered in formats such as CSV, JSON, XML, or via APIs, enabling seamless integration into your systems. Delivery frequencies range from real-time updates to scheduled intervals (daily, weekly, monthly, or on-demand). Choose datasets that align with your preferred delivery method and system compatibility for Alternative Data.
How Much Does Alternative Data Cost?
The cost of Alternative Data depends on factors like the datasets size, scope, update frequency, and customization level. Pricing models may include one-off purchases, monthly or yearly subscriptions, or usage-based fees. Many providers offer free samples, allowing you to evaluate the suitability of Alternative Data for your needs.
What Are Similar Data Types to Alternative Data?
Alternative Data is similar to other data types, such as Stock Market Data, ESG Data, Credit Rating Data, Commodity Data, and Currency Data. These related categories are often used together for applications like Hedge Funds and Algorithmic Trading.
- Overview
- Datasets
- Providers
- Use Cases
- Attributes
- Guide
- FAQ