Retail Banking Datasets for Transaction Data Analysis
Retail banking datasets refer to a collection of structured and organized data related to various aspects of retail banking operations. These datasets typically include information about customer demographics, account details, transaction history, loan and credit card details, customer interactions, and other relevant data points. The purpose of retail banking datasets is to provide insights and analysis for banks and financial institutions to better understand their customers, improve their products and services, and make informed business decisions. By analyzing these datasets, banks can identify patterns, trends, and customer behavior, which can help them personalize their offerings, detect fraud, manage risk, and optimize their operations. Some common examples of retail banking datasets include customer profiles, transactional data, credit scores, loan repayment history, customer feedback, and market research data. These datasets are often used for various purposes such as customer segmentation, risk assessment, marketing campaigns, product development, and compliance with regulatory requirements. Overall, retail banking datasets play a crucial role in enabling banks to leverage data-driven insights to enhance customer experience, drive profitability, and stay competitive in the rapidly evolving banking industry.
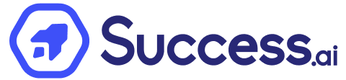
Company Financial Data | Banking & Capital Markets Professionals in the Middle East | Verified Global Profiles from 700M+ Dataset

Bank Data | Top 5 Largest Banks & ATMs in US | Location Data | Places Data

Global Bank Location and Visitation Metrics

Consumer Transaction Data | UK & FR | 600K+ daily active users | Retail - Footwear | Raw, Aggregated & Ticker Level

B2B Email Data | 100% Verified Business Email Address | 181M+ Contacts | (Direct Dails) | Decision Makers Direct Emails | 16+ Attributes

Envestnet | Yodlee's De-Identified Bank Statement Research Panel | USA Employee Payroll Data covering 4800+ employers | Cohort Analysis

Germany Point of Interest (POI) and Visitation Data| 7.5M+ Businesses

PREDIK Data-Driven I Point of Interest (POI) Data + Foot Traffic Data I +60 Data Variables for the LATAM Market

Brand Scorecard – Service: Consumer experience, engagement, and retention metrics for evaluating service brand performance

190K Weekly Movers, Pre-Movers & Under Contract MLS Postal Mailing List | Home Ownership Data
What is transaction data analysis in retail banking?
Transaction data analysis in retail banking refers to the process of analyzing and interpreting the vast amount of data generated from customer transactions. It involves extracting valuable insights and patterns from this data to understand customer behavior, preferences, and trends. By analyzing transaction data, banks can make informed decisions, improve customer experience, detect fraud, and develop targeted marketing strategies.
Why is transaction data analysis important for retail banks?
Transaction data analysis is crucial for retail banks as it provides valuable insights into customer behavior and preferences. By analyzing transaction data, banks can identify patterns and trends, which can help them personalize their services, improve customer satisfaction, and increase customer loyalty. Additionally, transaction data analysis enables banks to detect and prevent fraudulent activities, enhance risk management, and optimize operational efficiency.
What are the best retail banking datasets for transaction data analysis?
-
Customer transaction history: This dataset includes detailed information about customer transactions, such as date, time, transaction type, amount, and location. It provides a comprehensive view of customer behavior and can be used to identify spending patterns, predict future transactions, and segment customers based on their transaction history.
-
Merchant data: Merchant data contains information about the businesses where customers make transactions. It includes details such as merchant name, industry, location, and transaction volume. Analyzing this dataset can help banks understand customer preferences, identify popular merchants, and develop targeted marketing campaigns.
-
Demographic data: Demographic data includes information about customers’ age, gender, income, occupation, and location. By combining transaction data with demographic data, banks can gain insights into the spending habits and preferences of different customer segments. This information can be used to personalize offers, tailor marketing strategies, and improve customer segmentation.
-
Fraud detection data: Fraud detection data consists of information related to fraudulent activities, such as suspicious transactions, chargebacks, and identity theft. Analyzing this dataset helps banks identify patterns and anomalies that indicate fraudulent behavior, enabling them to take proactive measures to prevent fraud and protect their customers.