What is Textual Data? Explore AI & ML Training Datasets
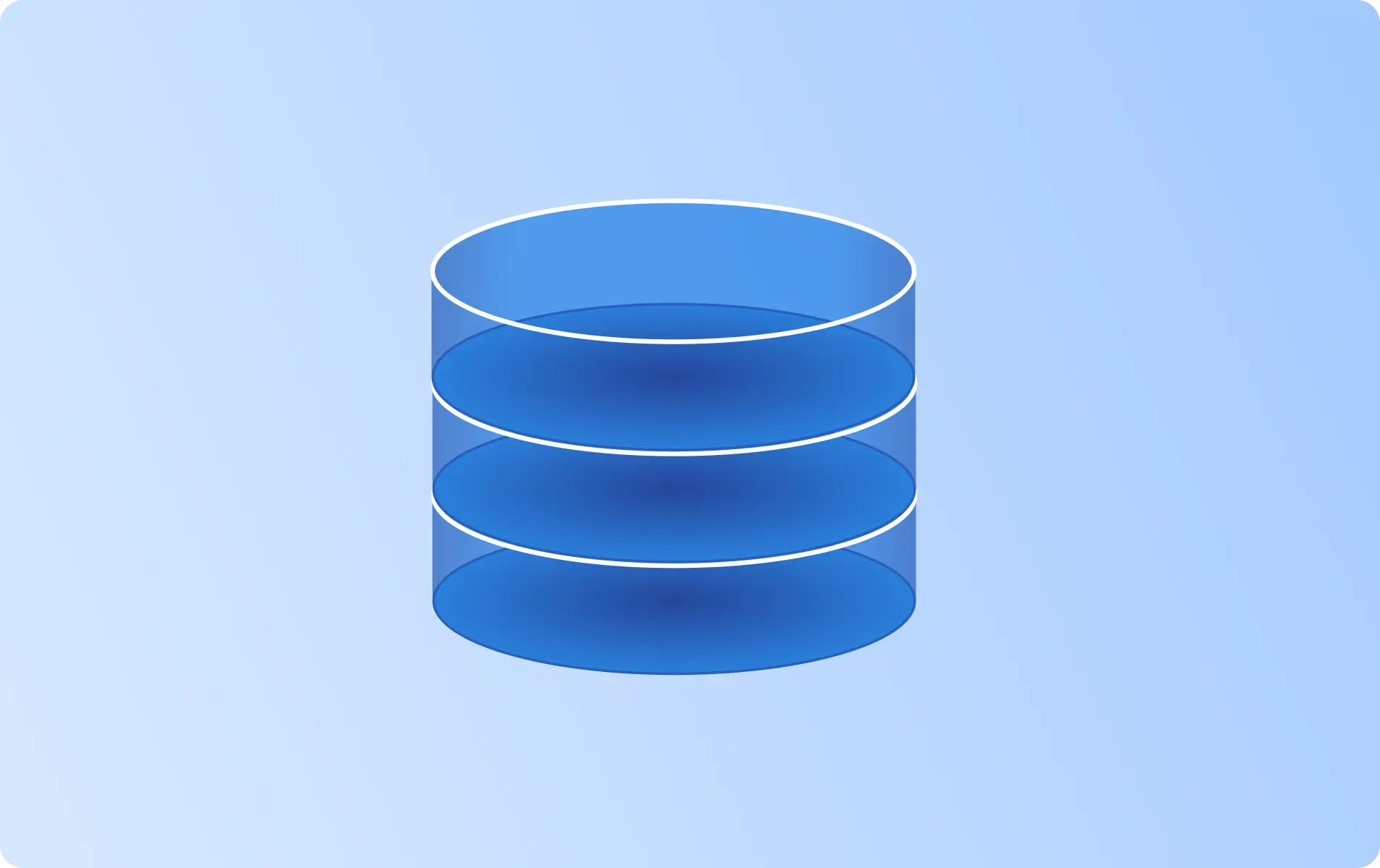
What is Textual Data?
Textual data refers to information that is expressed in words and numbers in written, digital or printed form. Most common examples of textual data include articles, books, chat messages, emails, blog posts, social media posts, forum comments, reviews, audio and video transcripts. Textual data plays a crucial role in developing AI models, training machine learning algorithms, and processing natural language. On this page, you’ll understand why textual data is important, its uses, and examples of the best applications.
Best Textual data Datasets & APIs
Textual Data | NLP-enriched Data | Transcription Data | Entity Extraction & Disambiguation | Ready-to-use
FileMarket | Text Recognition Data | 50,000 Images | Computer Vision Data | AI Model Training Data | Textual data | Annotated Imagery Data
Textual Data API | Deep Learning Data | Full Text | Firehose | 3.5M+ daily news articles | Noise-free
PDF Scraping Textual Data | Transcription Data | E-Receipt Data, PDF Text Extraction
Bitext | AI Training Data | Textual Data | 9 Languages for Synthetic Text Data | 100% Utterances Semantically Equivalent | 20 Verticals Covered
Factori AI & ML Training Data | Consumer Data | USA | Machine Learning Data
Coresignal | Clean Data | Company Data | AI-Enriched Datasets | Global / 35M+ Records / Updated Weekly
Nexdata | Multilingual Parallel Corpus Data | 200 Million Pairs | Text AI Training Data | Natural Language Processing Data | Translation Data
WebAutomation Off the Shelf Datasets | Audio Data for AI & ML Training | 600+ Hours of Recording | Speech Recognition, Natural Language Processing
Monetize data on Datarade Marketplace
Textual data Use Cases
What are Examples of Textual Data?
Textual data is the backbone of AI training data. This data can be found in various forms. Some examples include:
- Books: Includes novels, poems, and nonfiction.
- Articles and Academic Papers: Writings in academic journals and at conferences.
- News Articles: Texts from newspapers and online sources.
- Social Media Posts: Content from platforms like X, Facebook, Instagram, and blogs, including comments.
- Emails and Letters: Personal and professional communication.
- Translations: Texts converted from one language to another.
- Legal Documents: Contracts, court and legislative texts, and rulings.
- Medical Records: Patient records, doctors’ notes, and health documents.
- Corporate Documents: Reports, memos, and business plans.
- Government Publications: Public records and policy documents.
- Interview Transcripts: Written records of verbal interactions.
- Product Reviews: Reviews and feedback provided by customers on various platforms.
- Chat and Text Message: Conversations from messaging apps and customer service chats.
What are the Different Types of Textual Data?
Textual data can be categorized into various types based on its structure and format. Here are some of the primary types of textual data:
1. Structured Text
- Tabular Data: Text in a structured format like spreadsheets or databases, where information is organized into rows and columns.
- Logs and System Outputs: Data from software logs or system monitoring tools, often structured with timestamps and error codes.
2. Unstructured Text
- Social Media Posts: Text from platforms like Twitter, Facebook, and Instagram, including posts, comments, and messages.
- Emails and Messages: Communication data from emails, SMS, and chat applications.
- Articles and News: Text from online articles, blog posts, and news websites.
- Books and Literature: Digital texts of books, novels, and other literary works.
3. Semi-Structured Text
- HTML and XML Files: Web pages and documents that contain text with tags and metadata.
- JSON and YAML Data: Data interchange formats used to structure data for easy reading and writing by machines.
4. Speech-to-Text Data
- Transcriptions: Text obtained from converting spoken language into written form, such as transcripts from interviews, podcasts, and meetings.
- Voice Commands: Text derived from voice inputs used in virtual assistants and speech recognition systems.
5. Text from Digital Interactions
- Reviews and Feedback: User-generated content from product reviews, feedback forms, and surveys.
- Customer Support Interactions: Text from chat logs, support tickets, and customer service emails.
What are the Most Popular Uses for Textual Data?
Textual data serves numerous applications across industries. Here’s a look at some of the most prominent uses:
- Large Language Model (LLM) Training: Training models like OpenAI’s GPT series on vast text corpora to generate human language at an advanced level.
- Natural Language Processing (NLP): Includes tasks like sentiment analysis, where text is assessed to identify sentiments—positive, negative, or neutral.
- Named Entity Recognition (NER): Detecting and categorizing entities such as names, dates, and locations within texts.
- Translation: Translating languages in text, such as converting English to Spanish.
- Question Answering Systems: Creating systems capable of answering questions based on text data, akin to virtual assistants like Siri or Alexa.
- Automated Text Generation: Generating human-like text for chatbots, content creation, and interactive narratives.
- Text Summarization: Producing brief summaries of lengthy documents, useful for news and academic papers.
- Spam Detection: Classifying messages or emails as spam or not. This enhances email security.
- Topic Modeling: Identifying core themes within a collection of documents.
What is Textual Data in Machine Learning?
Textual data in machine learning refers to information extracted from written or spoken language, used to train algorithms for tasks like Natural Language Processing (NLP), sentiment analysis, and text classification. Working with textual data is a core aspect of NLP, which focuses on developing methods for computers to understand and respond to human language.
What is Textual Data analysis?
Textual data analysis enables researchers and analysts to make sense of large volumes of text and extract actionable insight. This analysis can be either qualitative or quantitative, depending on the data and research objectives.
Types of Textual Data Analysis
Qualitative Analysis
This analysis focuses on understanding the content, context, and meanings within texts. Techniques include:
- Content Analysis: This involves the identification and quantification of certain words or phrases to understand their frequency and relevance.
- Thematic Analysis: This technique extracts themes or concepts to reveal the underlying ideas in a text.
- Discourse Analysis: This is the study of how language is used in texts to reveal social norms, power dynamics, and ideological commitments.
- Narrative Analysis: This involves examining stories or narratives in texts to understand how they are constructed and what they communicate about reality.
Quantitative Analysis
This analysis uses statistical methods to convert text into data that can be quantified to discover patterns and trends. Techniques include:
- Word Frequency Count: This measures the most commonly used words or terms to assess the focus or emphasis of the text.
- Sentiment Analysis: This uses algorithms to identify and categorize opinions expressed in text data to determine the writer’s attitude.
- Text Classification: This involves assigning categories to text based on its content, such as detecting spam in emails.
- Overview
- Datasets
- Providers
- Use Cases
- Guide