Best Conversational AI Dataset for Natural Language Processing
Conversational AI datasets refer to collections of data specifically designed to train and improve conversational artificial intelligence systems. These datasets consist of various types of conversational interactions, such as text-based conversations, chat logs, or voice recordings, which are used to teach AI models how to understand and respond to human language. By leveraging these datasets, developers and researchers can enhance the natural language processing capabilities of AI systems, enabling them to engage in more human-like conversations and provide accurate and contextually relevant responses. Conversational AI datasets are crucial for advancing the field of AI and enabling the development of intelligent virtual assistants, chatbots, and other conversational applications.

Shaip - Multilingual Conversational AI Training Data (Text & Audio)
FileMarket | 20,000 Voice Memos | Multilingual Training Data for Conversational AI | Machine Learning (ML) Data

16kHz Conversational Speech Data | 35,000 Hours | Large Language Model(LLM) Data | Speech AI Datasets|Machine Learning (ML) Data
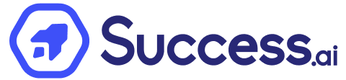
Success.ai | B2B Buyer Intent Data – 15,000+ Intent Topics Real-Time Insights – Global – Best Price & Quality Guarantee & AI-verified Records

Machine Learning (ML) Data | 800M+ B2B Profiles | AI-Ready for Deep Learning (DL), NLP & LLM Training
Related searches

AI Training Data | US Transcription Data| Unique Consumer Sentiment Data: Transcription of the calls to the companies

B2B Email Data | 230+ Million Verified Personal & Business B2B Email Data | Global Email Coverage

8kHz Conversational Speech Data | 15,000 Hours | Audio Data | Speech Recognition Data| Machine Learning (ML) Data
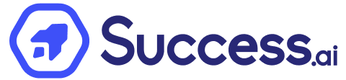
Success.ai | Purchase Intent Data | 15,000+ Intent Topics – Best Price Guarantee

Email Address Data | 230+ Million Verified Personal & Business Email Address Data | Global Email Coverage
What are conversational AI datasets?
Conversational AI datasets refer to collections of data specifically designed to train and improve conversational artificial intelligence systems. These datasets consist of various types of conversational interactions, such as text-based conversations, chat logs, or voice recordings, which are used to teach AI models how to understand and respond to human language.
How are conversational AI datasets used?
Conversational AI datasets are used to train AI models and improve their natural language processing capabilities. By analyzing the data in these datasets, AI systems can learn patterns, understand context, and generate accurate and contextually relevant responses in conversations with humans.
What types of data are included in conversational AI datasets?
Conversational AI datasets can include various types of data, such as text-based conversations, chat logs, voice recordings, and metadata. These datasets may also contain additional information, such as user demographics, timestamps, or annotations, to provide more context for training AI models.
Why are conversational AI datasets important?
Conversational AI datasets are crucial for advancing the field of AI and enabling the development of intelligent virtual assistants, chatbots, and other conversational applications. These datasets help improve the accuracy and effectiveness of AI systems in understanding and responding to human language, making them more useful and engaging for users.
Where can I find conversational AI datasets?
Conversational AI datasets can be found in various sources, including academic research repositories, open data initiatives, and commercial platforms. Some popular sources for conversational AI datasets include the Stanford Dialogue Corpus, the Persona-Chat dataset, and the Microsoft Research Open Data project.
How can I contribute to conversational AI datasets?
If you have relevant conversational data that you would like to contribute, you can reach out to the creators or maintainers of existing conversational AI datasets. They may have guidelines or processes in place for accepting contributions or may be interested in collaborating on new dataset creation initiatives.