10 Essential Diabetes Datasets for Research and Analysis
Diabetes datasets are collections of data related to diabetes, a chronic condition characterized by high blood sugar levels. These datasets typically include information such as patient demographics, medical history, laboratory test results, medication usage, and other relevant variables. These datasets are used for research, analysis, and development of predictive models to better understand and manage diabetes.
Recommended Diabetes Datasets

Ailment & Terminal Illness Leads & Data | 130 MM Ailment Records | Healthcare Contact Data

Hospitals and Nursing Database/ Hospitals and Nursing Data( With email, phone and NPI).

Global B2B Healthcare Professionals Data | 16 MM Mailing List Masterfile

US Pharmacists Email Database |Pharmacy Contact Data Lists( With phone, email,NPI)

US Healthcare, Doctor and phyisicians professionals Database ( With NPI's)
Related searches

Healthcare Marketing Data/ B2b Healthcare leads (With email, NPI's and phone )

DXRX by Diaceutics - Lab Mapping & Testing, Global Data

DXRX by Diaceutics - RW Testing & Lab Data Globally

DXRX by Diaceutics - Physician Mapping - US Data

Diaceutics Repository of Diagnostic Testing Data
Can't find the data you're looking for?
Let data providers come to you by posting your request
Post your request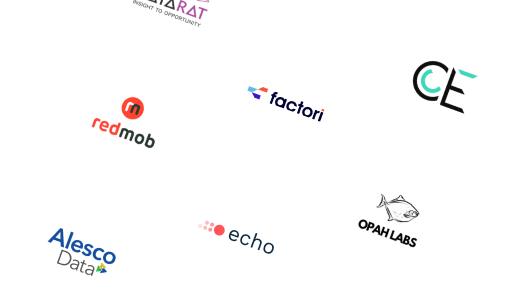
1. What are diabetes datasets?
Diabetes datasets are collections of structured data that contain information related to diabetes, such as patient demographics, medical records, laboratory test results, and other relevant variables. These datasets are used for research and analysis purposes to gain insights into diabetes, its causes, treatments, and outcomes.
2. Why are diabetes datasets important for research and analysis?
Diabetes datasets play a crucial role in advancing our understanding of diabetes and improving patient care. By analyzing these datasets, researchers can identify patterns, trends, and risk factors associated with diabetes. They can also evaluate the effectiveness of different treatments, interventions, and preventive measures. Diabetes datasets provide a valuable resource for evidence-based decision-making and the development of new strategies to combat diabetes.
3. How were the top 10 diabetes datasets selected?
The top 10 diabetes datasets were selected based on their relevance, quality, and availability for research and analysis purposes. These datasets have been widely used by researchers in the field of diabetes and have contributed significantly to scientific advancements. The selection process involved considering factors such as dataset size, diversity of variables, data quality, and the reputation of the source or organization providing the dataset.
4. Can I access and use the top 10 diabetes datasets for my research?
Yes, most of the top 10 diabetes datasets are publicly available and can be accessed for research purposes. However, it is important to review the terms and conditions of each dataset to ensure compliance with any usage restrictions or licensing requirements. Some datasets may require registration or approval before access is granted. Additionally, it is good practice to acknowledge the source of the dataset in any publications or research outputs.
5. Are the top 10 diabetes datasets suitable for analysis by researchers with different levels of expertise?
Yes, the top 10 diabetes datasets cater to researchers with varying levels of expertise. While some datasets may require advanced statistical or data analysis skills, others are designed to be more accessible to researchers with basic knowledge in the field. It is recommended to review the documentation and accompanying resources provided with each dataset to determine its suitability for your specific research needs and expertise.
6. Can I combine multiple diabetes datasets for my research and analysis?
In many cases, it is possible to combine multiple diabetes datasets to enhance the scope and depth of your research and analysis. However, it is important to ensure compatibility and consistency in data formats, variables, and data collection methods when merging datasets.