Top OCR Datasets for Precise Text Recognition
OCR datasets, or Optical Character Recognition datasets, are collections of images or documents that are used to train and evaluate OCR systems. These datasets typically contain a variety of text samples in different languages, fonts, and styles. They are labeled with the corresponding ground truth text to enable the training of machine learning models to accurately recognize and extract text from images or scanned documents. OCR datasets are crucial for developing and improving OCR algorithms and applications.
Recommended Ocr Datasets
Pixta AI | Imagery Data | Global | 5,000 Stock Images | Annotation and Labelling Services Provided | Japanese OCR images in nature scenes for AI & ML
DocuTrie| Receipt Data | AI-OCR for Document Processing | Bills, Invoices, Receipts and more with updated and custom data templates
Knuckle Head OCR Invoice Images Dataset - available for several industries in USA & India
TagX | 10000+ Multilingual Image Dataset | Text Detection | Global coverage | LLM data | LLM finetuning
SemanticForce Global News Data Monitoring | All Languages | Global Coverage | 100K+ e-News Sites | 2M articles daily | Archive Data & Real-Time
Related searches
SemanticForce’s Reddit Brand/Company/Product/Person Mention and Sentiment
Airbnb Data by State - Active listings count, average daily rate, average occupancy [USA] [SEP 24TH-OCT 24TH] [2021]
Airbnb Data by County - Active listings count, average daily rate, average occupancy [USA] [SEP 24TH-OCT 24TH] [2021]
Airbnb Data by Zipcode - Active listings count, average daily rate, average occupancy [USA] [SEP 24TH-OCT 24TH] [2021]
Can't find the data you're looking for?
Let data providers come to you by posting your request
Post your request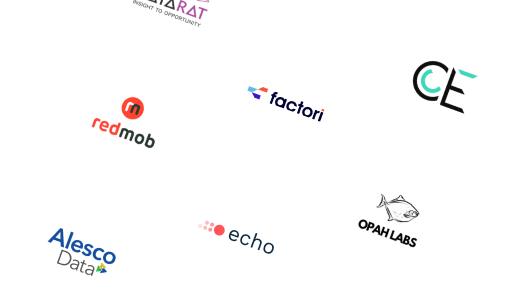
1. What is OCR?
OCR stands for Optical Character Recognition. It is a technology that enables the conversion of printed or handwritten text into machine-readable text. OCR systems use various techniques to analyze and interpret characters, allowing for accurate text recognition.
2. Why is accurate text recognition important?
Accurate text recognition is crucial for a wide range of applications, including document digitization, data extraction, text-to-speech conversion, and language translation. It enables efficient processing and analysis of textual information, saving time and effort in manual data entry tasks.
3. What are OCR datasets?
OCR datasets are collections of images or documents that are specifically curated for training and evaluating OCR systems. These datasets contain a variety of text samples with different fonts, sizes, orientations, and backgrounds. They serve as a benchmark for measuring the accuracy and performance of OCR algorithms.
4. How do OCR datasets help improve text recognition accuracy?
OCR datasets provide a diverse set of text samples that cover various real-world scenarios. By training OCR models on these datasets, developers can improve the algorithms’ ability to handle different fonts, languages, and document layouts. Additionally, OCR datasets allow for benchmarking and comparing the performance of different OCR systems.
5. What are some popular OCR datasets?
Some popular OCR datasets include:
- MNIST: A widely used dataset for handwritten digit recognition, which can be adapted for OCR tasks.
- IAM Handwriting Database: Contains handwritten English text samples for training and evaluating OCR systems.
- RVL-CDIP: A dataset with a large collection of scanned documents from various sources, suitable for OCR research.
- COCO-Text: An image dataset that includes text annotations, useful for OCR in natural scene images.
- SynthText: A dataset that generates synthetic images with text annotations, allowing for large-scale OCR training.