What is Object Detection Data? Uses, Types & Data Examples
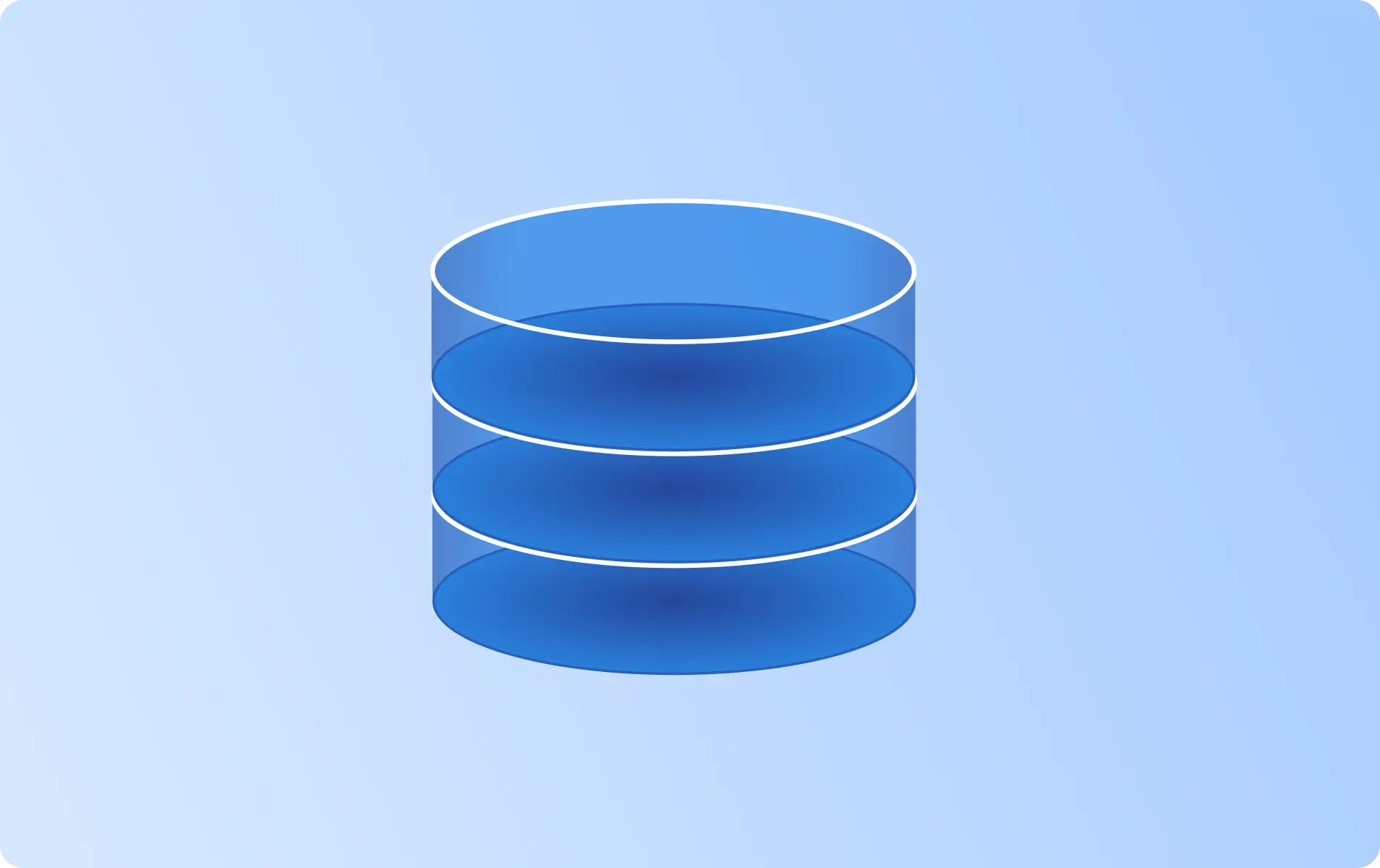
- Overview
- Datasets
- Providers
- Use Cases
- Attributes
- Guide
- FAQ
- Overview
- Datasets
- Providers
- Use Cases
- Attributes
- Guide
- FAQ
What is Object Detection Data?
Object detection data is a collection of images or videos annotated with labels indicating the presence and location of various objects. It consists of data with visual information marked by bounding boxes, segmentation masks, or key points. On this page, you’ll find the best data sources for different types of object detection data.
Best Object Detection Databases & Datasets
Here is our curated selection of top Object Detection Data sources. We focus on key factors such as data reliability, accuracy, and flexibility to meet diverse use-case requirements. These datasets are provided by trusted providers known for delivering high-quality, up-to-date information.

600K+ Household Object Images | AI Training Data | Object Detection Data | Annotated imagery data | Global Coverage

Annotated Imagery Data |Object Detection Data| AI Training Data| Car images | 100,000 Stock Images

Furniture Object Detection Dataset | Multi-Angle AI Training | 100% Global Coverage

Driver Technologies | Car Object Detection Video Data | North America and UK | Real-time and historical traffic information

340K+ Jewelry Images | AI Training Data | Object Detection Data | Annotated imagery data | Global Coverage

Imagery Data | Object Detection Data| AI Training Data| E-commerce apparel dataset | 10,000 Stock Images

OORT - Object Detection Data | Diverse Home Repair Tools Dataset for Machine Learning | 125 Categories, 100K+ Data Points

B2B Data | Global Technographic Data | Sourced from HTML, Java Scripts & Jobs | 921M+ Records

FileMarket | 20,000 photos | AI Training Data | Large Language Model (LLM) Data | Machine Learning (ML) Data | Deep Learning (DL) Data |

Driver Technologies | Truck Object Detection Video Data | North America and UK | Real-time and historical traffic information
Can't find the data you're looking for?
Let data providers come to you by posting your request
Post your request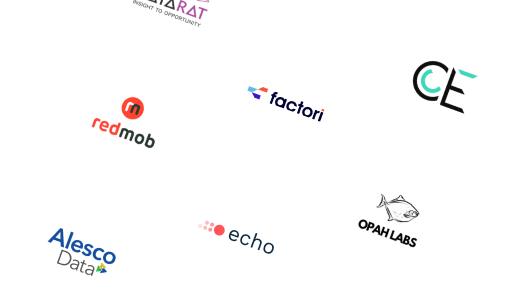
Top Object Detection Data Providers & Companies
Popular Use Cases for Object Detection Data
Object Detection Data is essential for a wide range of business applications, offering valuable insights and driving opportunities across industries. Below, we have highlighted the most significant use cases for Object Detection Data.
Main Attributes of Object Detection Data
Below, we outline the most popular attributes associated with this type of data—features that data buyers are actively seeking to meet their needs.
Attribute | Type | Description | Action |
---|---|---|---|
Float | The latitude of a point on earth's surface. Commonly abbreviated as "lat". | View 23 datasets | |
String | Mobile Ad ID is a sequence of random symbols, given by the mobile device’s operating system. It’s shared with the servers of the apps that the user is using to track his customer journey and “remember” his or her choices. | View 12 datasets | |
String | The country code in Alpha-2 format (ISO 3166) | View 5 datasets | |
Float | The longitude of a point on earth's surface. Commonly abbreviated as "long". | View 4 datasets | |
String | The name of a point of interest (POI). | View 3 datasets | |
Integer | The age of a contact. | View 1 datasets |
What are Examples of Object Detection Data?
Examples include labeled images for autonomous vehicles, annotated videos for security purposes, and tagged pictures for retail analytics. This data is crucial for training machine learning models to detect and identify objects across various scenarios.
What Type of Data is Required for Object Detection Data?
Data required for object detection includes images and videos that are annotated to indicate the presence and location of objects. This data needs to be:
- Labeled with Bounding Boxes: To identify the exact location of objects.
- Segmented with Masks: For more detailed object boundaries.
- Annotated with Key Points: For specific object features.
How is Object Detection Data Collected?
Collecting data for object detection involves several steps:
- Data Acquisition: Gathering images and videos from various sources like cameras, online repositories, or synthetic generation. Synthetic data generation is also a valuable method for expanding datasets.
- Annotation: Manually or automatically labeling the data with bounding boxes, segmentation masks, or key points.
- Data Augmentation: Applying techniques like rotation, scaling, cropping, and flipping to images to create variations and improve diversity of the dataset.
What is the Format for Object Detection Data?
Object detection data is typically stored in formats that facilitate access and processing. Common formats include:
- XML or JSON: Used to store annotations, specifying the coordinates of bounding boxes and other relevant information.
- Image Files: Usually in JPEG or PNG formats for the visual data.
- TFRecord: A format used by TensorFlow that stores both images and annotations in a single file.
How Does Object Detection Data integrate with Other AI Training Data?
Object detection data can be integrated with other types of AI training data to enhance model performance. For instance, combining object detection data with textual data and annotated imagery data can provide a more context for machine learning models. This integration is useful in applications like autonomous driving, where the model needs to understand both visual and textual data.
How is Object Detection Data Used in AI?
Object detection data is used to train models that can identify and locate objects within images and videos. Applications include:
- Autonomous Vehicles: Detecting pedestrians, vehicles, and obstacles.
- Security Systems: Identifying suspicious activities or intrusions.
- Retail Analytics: Analyzing shopper behavior and product placement.
- Healthcare: Detecting anatomical structures and abnormalities in scans such MRIs.
Which Algorithm is Best for Object Detection Data?
Several algorithms are highly effective for object detection, each with its strengths:
- YOLO (You Only Look Once): Known for its speed and real-time object detection capabilities.
- Faster R-CNN: Offers high accuracy, suitable for applications requiring precise detection.
- SSD (Single Shot MultiBox Detector): Balances speed and accuracy, making it popular for various tasks.
- RetinaNet: Handles different object sizes and frequencies.
Frequently Asked Questions
How is the Quality of Object Detection Data Maintained?
The quality of Object Detection Data is ensured through rigorous validation processes, such as cross-referencing with reliable sources, monitoring accuracy rates, and filtering out inconsistencies. High-quality datasets often report match rates, regular updates, and adherence to industry standards.
How Frequently is Object Detection Data Updated?
The update frequency for Object Detection Data varies by provider and dataset. Some datasets are refreshed daily or weekly, while others update less frequently. When evaluating options, ensure you select a dataset with a frequency that suits your specific use case.
Is Object Detection Data Secure?
The security of Object Detection Data is prioritized through compliance with industry standards, including encryption, anonymization, and secure delivery methods like SFTP and APIs. At Datarade, we enforce strict policies, requiring all our providers to adhere to regulations such as GDPR, CCPA, and other relevant data protection standards.
How is Object Detection Data Delivered?
Object Detection Data can be delivered in formats such as CSV, JSON, XML, or via APIs, enabling seamless integration into your systems. Delivery frequencies range from real-time updates to scheduled intervals (daily, weekly, monthly, or on-demand). Choose datasets that align with your preferred delivery method and system compatibility for Object Detection Data.
How Much Does Object Detection Data Cost?
The cost of Object Detection Data depends on factors like the datasets size, scope, update frequency, and customization level. Pricing models may include one-off purchases, monthly or yearly subscriptions, or usage-based fees. Many providers offer free samples, allowing you to evaluate the suitability of Object Detection Data for your needs.
What Are Similar Data Types to Object Detection Data?
Object Detection Data is similar to other data types, such as Logo Data, Biometric Data, Medical Imaging Data, and Facial Recognition Data. These related categories are often used together for applications like Iris Diagnostics and Deep Learning Medical Imaging.