Top Text Datasets for Natural Language Processing
Text datasets are collections of textual data, such as articles, books, reviews, tweets, or any other form of written content. These datasets are used for various natural language processing (NLP) tasks, including text classification, sentiment analysis, machine translation, and more. Text datasets are essential for training and evaluating NLP models and algorithms.

Fine-Tuning Text Data | 2 Millions | User Generated Text |Foundation Model | SFT Data | Large Language Model(LLM) Data

TagX | 10000+ Multilingual Image Dataset | Text Detection | Global coverage | LLM data | LLM finetuning

100K+ Text Rich Images | AI Training Data | Annotated imagery data for AI | Object & Scene Detection | Global Coverage

Data Collection by Shaip: Text, Audio, Image, Video for AI & ML Training

Semantic Text Analytics as a service - Dandelion API
Related searches

AI & ML Training Data | Artificial Intelligence (AI) | Machine Learning (ML) Datasets | Deep Learning Datasets | Easy to Integrate | Free Sample

Logo Data | B2B Leads Data | Global Key Customers Logo Scanning for New Leads | 244M+ Connections
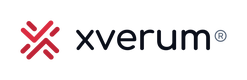
Nordic B2B Profiles Data | B2B Marketing Data | 10M Verified Leads for Norway, Sweden & Finland (100+ Attributes)

Keyboard Inputs Data APAC - Text, Voice and Search Queries (Conversation Intent & In-App Searches, 90M records) - 1st Party Data

Test Questions Data | 50 Millions | Foundation Model | Unsupervised Text Data | Large Language Model(LLM) Data
1. What is Natural Language Processing (NLP)?
Natural Language Processing (NLP) is a subfield of artificial intelligence (AI) that focuses on the interaction between computers and human language. It involves the development of algorithms and models to enable computers to understand, interpret, and generate human language in a way that is meaningful and useful.
2. Why are text datasets important for NLP?
Text datasets play a crucial role in training and evaluating NLP models. These datasets provide the necessary examples and patterns for machines to learn and understand human language. By using diverse and high-quality text datasets, NLP models can improve their performance in tasks such as text classification, sentiment analysis, machine translation, and more.
3. What makes a text dataset suitable for NLP?
A suitable text dataset for NLP should possess certain characteristics. It should be large enough to capture the complexity and diversity of human language. The dataset should also be well-annotated, meaning it has accurate labels or annotations that can be used for supervised learning. Additionally, a good text dataset should cover a wide range of topics and domains to ensure the model’s generalization capabilities.
4. Where can I find text datasets for NLP?
There are several reliable sources where you can find text datasets for NLP. Some popular options include academic research repositories, such as the Stanford NLP Group’s dataset collection, Kaggle, UCI Machine Learning Repository, and various government data portals. Additionally, many organizations and companies release their own datasets for public use, such as Google’s Natural Language Processing datasets.
5. What are some widely used text datasets for NLP?
There are numerous widely used text datasets for NLP, each serving different purposes. Some popular ones include the Gutenberg Books dataset, IMDb movie reviews dataset, Twitter sentiment analysis dataset, Wikipedia articles dataset, and the Amazon product reviews dataset. These datasets have been extensively used in research and benchmarking NLP models.
6. How can I evaluate the quality of a text dataset for NLP?
To evaluate the quality of a text dataset for NLP, you can consider several factors. Firstly, examine the dataset’s size and diversity to ensure it covers a wide range of language patterns. Secondly, check the dataset’s annotation quality and consistency. Additionally, it is important to assess the dataset’s relevance to your specific NLP task and the availability of a sufficient number of training examples.